In our blog post, ‘Why is Picking Apparel Hard for Most Robots', we discussed the difficulty of picking apparel for traditional automation due to:
- Packaging (e.g. transparent polybags)
- Tote packing (how loosely, tightly, or messily items are packed into a bin)
- Deformability (how much an item changes shape when picked up)
- Seasonal SKU rotation (the changing out of items seasonally)
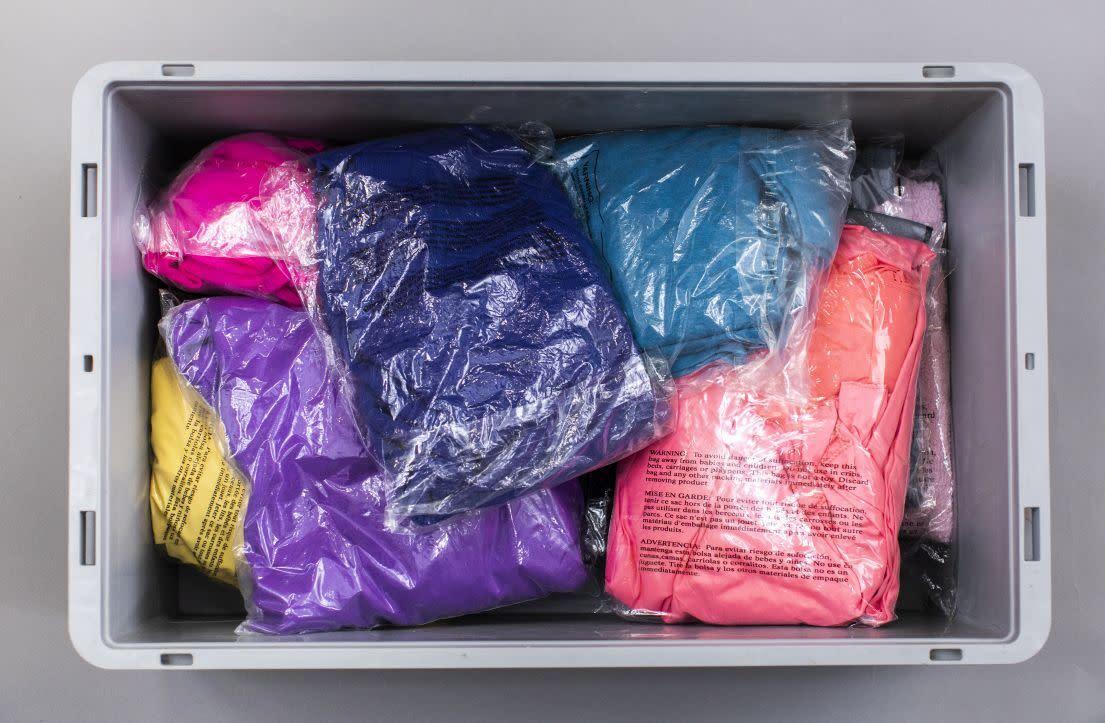
For traditional automation to handle apparel, every single variation (e.g. packaging, size, shape) needs to be hardcoded. This could mean literally millions of combinations and permutations. Add to this the seasonal rotation of SKUs, especially in fast fashion, and the complexity grows exponentially. The good news is that there is a solution that addresses these challenges to allow robots to pick virtually any apparel item, even ones the robots have never seen before: the Covariant Brain — our AI Robotics platform that enables robots to see, think, and act. How? The secret is in the way the Covariant Brain is specially designed to handle large variations and unstructured environments.
AI at the core of handling apparel
Robots powered by the Covariant Brain can handle virtually any item and unstructured scenario, even if they’ve seen neither before. To understand why this is, think about how humans pick and place items as opposed to the approaches that traditional automation takes: we see an item in a tote, pick it up, and place it where it needs to go. Seems easy, but doing this requires us to rapidly process visual information, intuit what grasp and how much force to use, and think through how to accurately transport and place that item into a tote or onto a moving conveyor belt. The instant that is done, the same process must happen for a completely new item with totally new dimensions. That takes complicated reasoning and cognition. How do we do it if no one explicitly hard-coded our neurons to do so? The answer is that we learned by doing throughout the course of our lifetimes. As babies, we grasped items too tightly and broke them. We picked up jars from the wrong end and spilled beads all over the floor. We reached for a rattle dozens of times before finally getting the trajectory right. Hardcoding of traditional automation means that there is only a finite number of things it can do, items it can recognize, and problems it can solve: you can explicitly program your robot to pick up a specific rattle in a specific number of ways, but likely not for any type of rattle in every different way. The Covariant Brain, on the other hand, infers from past experiences, learning through trial and error, in a manner much more reminiscent of how humans learn. In fact, the Covariant Brain thrives when given the unstructured, varied input that items like apparel present in order to learn, adapt, and grow – by design, it needs all of that variability to gain experiences to infer upon. It’s this adaptability that allows robots powered by the Covariant Brain, to:
- Perform autonomously out of the box
- Learn continuously, from robots in warehouses around the world
- Adapt in real-time to achieve success
This is a vast improvement on traditional automation. It means that Covariant-powered robots are effective and efficient when picking and placing in the highly variable environments found in warehouses and fulfillment centers handling apparel items.
Hardware designed for apparel
When it comes to handling apparel, Covariant robots combine modern AI with innovative solution design to achieve optimal performance and accuracy. Some examples of such solution design elements include apparel-specific grippers and item transport mechanisms.
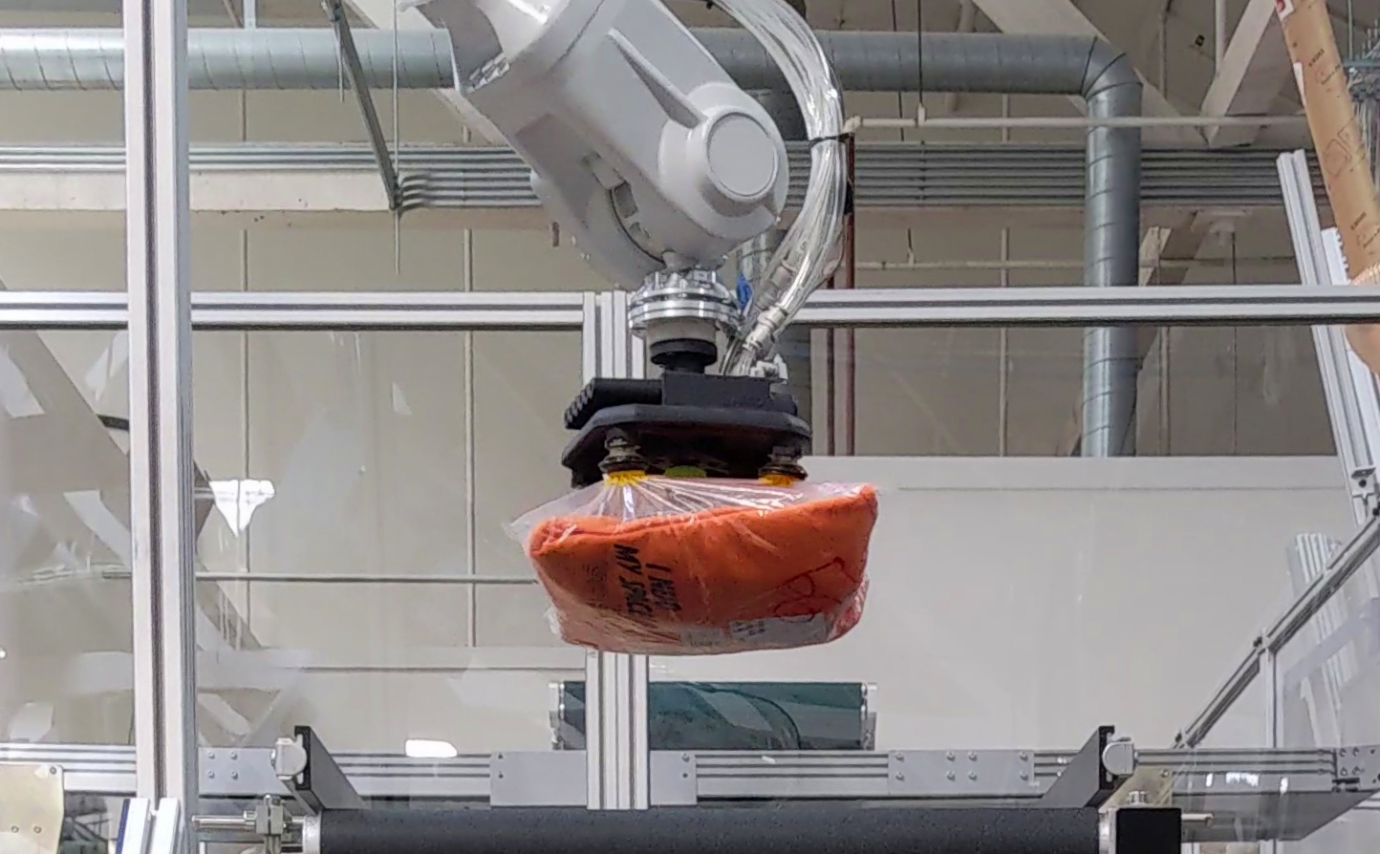
Adaptive suction cup grippers
One of the most important considerations when picking apparel is gripper design. For instance, Covariant robots use multiple vacuum-powered suction cups to allow for a diversity of grasps based on an apparel item’s physical characteristics (e.g. size, packaging, deformability). Each cup is individually actuated — meaning, the suction for each cup can be turned on or off individually. This is how a Covariant robot can go from handling something light and small, such as a glove, to something heavier and stiff, such as a jacket: it simply turns more cups on or keeps them off. (Note that outside of apparel, this still applies: a Covariant-powered robot can go from gripping lipstick to gripping a big box without flinching).
Sign up for our newsletter
Get the latest news in AI Robotics, picked just for you
Precise transport of items
Picking and placing require precise motion and path planning. Due to their deformability and varied size and weight, apparel items, in particular, must be carefully transported to their destination (e.g. unit sorter, putwall, order tote). Doing this quickly and accurately can be difficult both for humans and for traditional robots over long periods of time. Innovative solution design further helps maximize speed while minimizing the chances for error.
For example, when inducting items directly onto a unit sorter, such as a bomb bay or tilt tray sorter, items might need to be placed centered so they don’t jam the sorting mechanism further down the line. This can be difficult with fast-moving sorters. In the Covariant induction solution for fast-moving sorters, one novel design to increase performance is to have the robot place items onto an accelerator mounted above the sorter. The accelerator then places the item precisely in the center of the next available tray on the sorter as flatly as possible. Another example can be found in the design of the Covariant transporter putwall used for sorting batch-picked apparel items into individual orders. The robot arm picks up an apparel item from a batch-picked tote, scans it, and places it onto a series of conveyors, elevators, and linear transporters to deliver the item very quickly to the corresponding order cubby.
Robots are in fashion!
It wouldn’t make sense for a human to start from scratch every time they learned to pick up something new – and it doesn’t make sense for robots, either. With the help of neural nets and deep learning, Covariant-powered robots learn from their experience, approaching unknown and unstructured scenes with all of the knowledge of every scene they’ve encountered previously. This knowledge and flexibility mean that Covariant-powered robots and all of the powerful features they support are just what’s needed to pick and place within industries with highly variable SKUs. Or in other words: Covariant-powered robots are really, really good at picking shirts (and pants and jackets and whatever the newest fashion trend says is cool).