Foundation models have transformed artificial intelligence. The growth trajectory of robotics foundation models is accelerating rapidly thanks to access to large, highly diverse, production-setting robotic data. As a result, Covariant has invested in the next generation of AI technologies such as NVIDIA A100 Tensor Core and H100 Tensor Core GPUs for training and RTX A5000 GPUs for inference to increase compute power— enabling unprecedented levels of scale, intelligence, and adaptability for our customers.
While the idea of artificial intelligence has existed since 1956, it wasn’t until 2012 that we saw a major inflection point. That’s when AlexNet, a pioneering convolutional neural network (CNN), opened the door for modern AI.
Powered by deep learning-based neural networks, it became possible to train AI models to perform very specific tasks like natural language processing (NLP), image recognition, and language translation.
The next significant breakthrough in modern AI came with the advent of GPT, or Generative Pre-trained Transformers. Leveraging vast amounts of data from the internet, the core technology of GPTs has enabled AI models to be more generalized.
Instead of having separate models for specific tasks such as NLP and image recognition, GPT-based AI can perform a wide variety of tasks using a single foundation model.
The role of foundation models
Foundation models are neural networks “pre-trained” on massive amounts of data without specific use cases in mind. As that generalized model is trained on a wider set of tasks, performance on each specific task gets better, because of ‘transfer learning’.
Foundation models have transformed AI in the digital world — powering large language models (LLMs) such as ChatGPT and DALL-E. We’ve seen foundation models enable these applications to understand and react to the most unusual situations with human-like dexterity.
The impact of foundation models will go far beyond text and image generation — and into the physical world. Many believe that foundation models will one day be the basis of all AI-powered software applications.
But when it comes to applications in the physical world, like robotics, these foundation models have, for good reason, taken a little longer to build.
Driving a major breakthrough with Robotics Foundation Models
In 2017, Pieter Abbeel, Rocky Duan, Tianhao Zhang, and I co-founded Covariant to build the first foundation model for robotics. Previously, we had pioneered robot learning, deep reinforcement learning, and deep generative models at OpenAI and UC Berkeley.
There had been some promising academic research in AI-powered robotics, but we felt there was a significant gap when it came to applying these advancements to real-world applications, such as robotic picking in warehouses.
When thinking about applications in the physical world, why did we start with warehouses? It was because these are the ideal environments for AI models — many have hundreds of thousands or even millions of different stock-keeping units (SKUs) flowing through at any given moment.
That means a picking robot faces virtually infinite variability in terms of the types of objects it deals with and how they’re presented. Additionally, for robots to be successful in real-world warehouses, they need to perform with high reliability and high flexibility. The Covariant Brain leverages our robotics foundation model to address all these challenges, allowing robots to perform autonomously in warehouses.
Proprietary real-world data at the core of Robotics Foundation Models
One key factor that has enabled the success of generative AI in the digital world is a foundation model trained on a tremendous amount of internet-scale data.
However, a comparable dataset did not previously exist in the physical world to train a robotics foundation model. That dataset had to be built from the ground up — composed of vast amounts of “warehouse-scale” real-world data and synthetic data.
So how did we build this dataset in a relatively short time? Covariant’s unique application of fleet learning enables hundreds of connected robots across 4 different continents to share live data and learnings across the entire fleet.
This real-world data has extremely high fidelity and surfaces new unknown factors that researchers would not have been able to imagine. Added to this is synthetic data that provides infinite variations of known factors. This data is multimodal, including images, depth maps, robot trajectories, and time-series suction readings.
Covariant’s robotics foundation model relies on this mix of data. General image data, paired with text data, is used to help the model learn a foundational semantic understanding of the visual world. Real-world warehouse production data, augmented with simulation data, is used to refine its understanding of specific tasks needed in warehouse operations, such as object identification, 3D understanding, grasp prediction, and place prediction.
Proprietary real-world data at the core of Robotics Foundation Models
One key factor that has enabled the success of generative AI in the digital world is a foundation model trained on a tremendous amount of internet-scale data.
However, a comparable dataset did not previously exist in the physical world to train a robotics foundation model. That dataset had to be built from the ground up — composed of vast amounts of “warehouse-scale” real-world data and synthetic data.
So how did we build this dataset in a relatively short time? Covariant’s unique application of fleet learning enables hundreds of connected robots across 4 different continents to share live data and learnings across the entire fleet.
This real-world data has extremely high fidelity and surfaces new unknown factors that researchers would not have been able to imagine. Added to this is synthetic data that provides infinite variations of known factors. This data is multimodal, including images, depth maps, robot trajectories, and time-series suction readings.
Covariant’s robotics foundation model relies on this mix of data. General image data, paired with text data, is used to help the model learn a foundational semantic understanding of the visual world. Real-world warehouse production data, augmented with simulation data, is used to refine its understanding of specific tasks needed in warehouse operations, such as object identification, 3D understanding, grasp prediction, and place prediction.
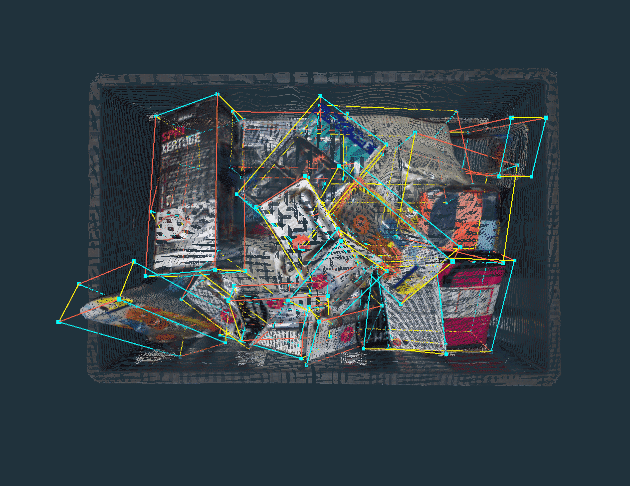
Pushing boundaries: Delivering real-world robotic autonomy at scale
As the amount of data grows and model complexity increases, Covariant has invested in the next generation of AI technologies to increase scalability and compute power. At the core of our compute power are NVIDIA A100 Tensor Core and H100 Tensor Core GPUs for training and RTX A5000 GPUs for inference.
With access to NVIDIA GPUs, Covariant’s world-class AI researchers and engineers can run hundreds of computing jobs simultaneously, instead of just a handful.
Covariant’s current robotics foundation model is unparalleled in terms of autonomy (level of reliability) and generality (breadth of supported hardware and use cases), and we’re continuing to further expand its reliability thanks to the unique data of (rare) long-tail errors we collect in production.
Autonomous robots, as they become more versatile and reliable, are making warehouses and fulfillment centers more efficient. They can perform tasks with greater accuracy, which pays dividends for consumers and businesses alike.
Most importantly, these advancements enable a future world where robots and people are working side by side. Robots handle dull, dangerous, and repetitive tasks while enabling the investment needed to create opportunities for meaningful work within environments that are critical to the economy but have struggled to maintain an engaged workforce.